
Importance of Customer Sentiment Analysis
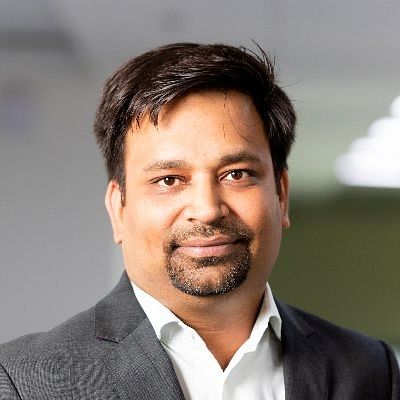
Customer sentiment analysis is a crucial tool for businesses to gauge and understand customer opinions, emotions, and attitudes towards their products, services, or brand. By analyzing customer sentiment, organizations can gather valuable insights to enhance their offerings, improve customer experiences, and make informed business decisions. In this blog, we will explore the importance of customer sentiment analysis, different types of sentiment analysis techniques, how to effectively analyze customer sentiment, and how Rezo.ai can assist in this process.
Why is Customer Sentiment Analysis Important?
Understanding customer sentiment is essential for several reasons. First and foremost, it enables businesses to gain deep insights into customer experiences and perceptions, allowing them to identify areas for improvement. By uncovering positive sentiments, companies can amplify and reinforce aspects of their products or services that customers appreciate. Conversely, detecting negative sentiments enables organizations to address dissatisfaction and prevent customer churn.
Customer sentiment analysis is also valuable for reputation management. By monitoring customer sentiment across various channels, such as social media, review sites, or customer surveys, businesses can promptly address negative feedback, resolve issues, and maintain a positive brand image.
Moreover, customer sentiment analysis helps companies anticipate market trends and stay ahead of the competition. By analyzing sentiment patterns, businesses can identify emerging needs and preferences, allowing them to adapt their strategies, develop new products or services, and meet customer demands.
Methods of Customer Sentiment Analysis
There are different methods of customer sentiment analysis techniques that businesses can employ:
- Manual Analysis: This approach involves manually reviewing and categorizing customer feedback, such as emails, comments, or reviews. While time-consuming, this method allows for in-depth analysis and understanding of customer sentiments.
- Automated Text Analysis: Leveraging natural language processing (NLP) and machine learning algorithms, automated text analysis tools can automatically analyze and categorize large volumes of unstructured customer data. This method provides more scalability and efficiency than manual analysis.
- Social Media Analysis: This technique focuses on extracting and analyzing customer sentiments expressed on social media platforms. By monitoring social media conversations, businesses can gain real-time insights into customer analysis, opinions, trends, and brand perception.
- Survey-Based Analysis: Using surveys or questionnaires, businesses collect direct feedback from customers, allowing for targeted sentiment analysis and specific insights into customer experiences.
Types of Customer Sentiment Analysis

Some common types of customer sentiment analysis include:
- Fine-grained analysis: This approach allows for a more nuanced understanding of sentiment by categorizing customer sentiments as very positive, positive, neutral, negative, or very negative.
- Aspect-based analysis: With this approach, customer sentiment is analyzed based on specific aspects or features of the product or service that are mentioned in the feedback. It helps identify sentiment towards each aspect individually.\Emotion detection: This type of analysis focuses on identifying and categorizing the emotions expressed by customers in their feedback, such as happiness, anger, sadness, or surprise.
- Emotion detection: This type of analysis focuses on identifying and categorizing the emotions expressed by customers in their feedback, such as happiness, anger, sadness, or surprise.
- Intent analysis: Intent analysis seeks to understand the intentions or underlying motivations behind customer feedback. It helps identify whether customers are expressing a desire for a particular product enhancement, reporting a problem, or seeking assistance.
Read More: How AI is Helping Enterprises in Building Customer Trust and Loyalty
How to collect data for customer sentiment analysis?
To collect data for customer sentiment analysis, businesses can employ various methods:
- Social Media Listening: Monitor mentions, hashtags, and conversations related to your brand on social media platforms.
- Review and Feedback Analysis: Analyze customer reviews, feedback forms, and comments on review sites, forums, or your website.
- Customer Surveys: Conduct surveys and questionnaires, asking customers to express their opinions, experiences, and suggestions.
- Call Center/Support Logs: Extract valuable insights from customer interactions with your call center or support team.
How to Analyze Customer Sentiment?
Once you have collected the data, it's time to analyze customer sentiment using the chosen technique:
- Preprocess the Data: Clean the data, remove noise, and categorize it based on relevant attributes.
- Apply Sentiment Analysis Techniques: Utilize manual or automated sentiment analysis tools to classify the data into positive, negative, or neutral sentiments.
- Quantify and Visualize the Results: Assign sentiment scores to each sentiment category and visualize the sentiment trends using charts or dashboards.
- Extract Actionable Insights: Interpret the results, identify patterns, and extract actionable insights to enhance the customer experience, address pain points, or improve products/services.
Read More: What is CSAT, How to Measure and Improve It
Customer Sentiment Analysis Use Case
To illustrate the practical application of customer sentiment analysis, let's consider a use case of a multinational hotel chain. By analyzing customer sentiment from online reviews, social media comments, and feedback surveys, the hotel chain identified recurring complaints about slow check-in processes. Armed with this insight, the company implemented measures to streamline check-in procedures, resulting in increased customer satisfaction and positive sentiment.
Leverage Rezo.ai for Customer Sentiment Analysis
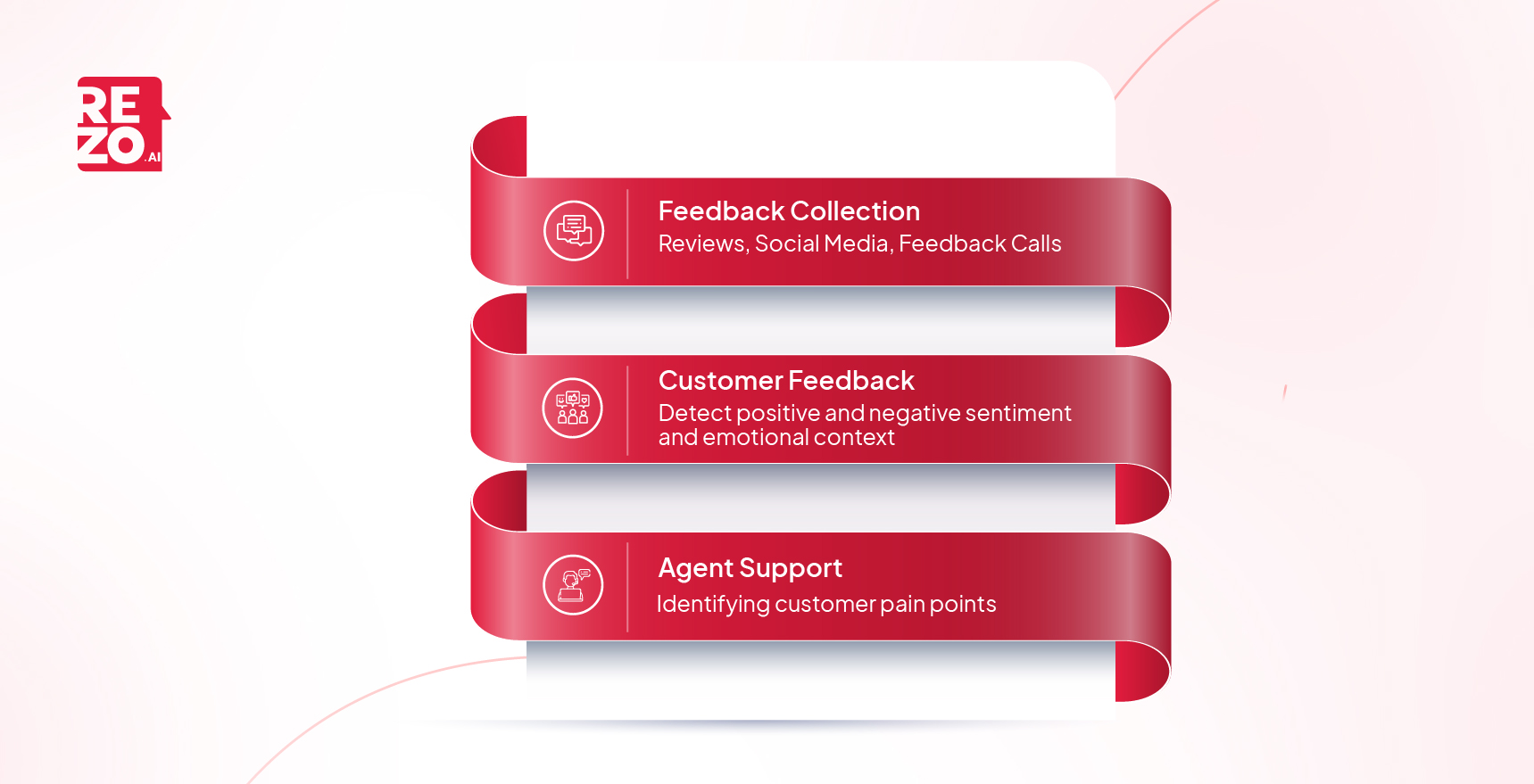
Leverage Rezo.ai for Customer Sentiment Analysis
At Rezo.AI, we believe that customer sentiment analysis is more than just understanding how customers feel about your brand—it's about uncovering actionable insights that drive better customer experiences. Our innovative AI-powered solution empowers businesses to tap into the true voice of their customers, revolutionizing the way they understand and engage with their audience.
By analyzing customer feedback, reviews, social media posts, and other sources of data, our cutting-edge sentiment analysis technology goes beyond surface-level sentiments. We delve deep into the nuances of customer opinions, uncovering valuable insights that help businesses make informed decisions and drive positive outcomes.
Imagine being able to identify and address customer pain points in real-time. With Rezo.AI's sentiment analysis, you gain real-time visibility into customer sentiments, allowing you to proactively resolve issues and provide tailored experiences that exceed expectations.
Our AI algorithms detect not just positive and negative sentiment, but also the underlying emotions expressed by customers. This helps you understand the emotional context behind their feedback, enabling you to connect with them on a more personal level. Whether it's happiness, frustration, or confusion, our sentiment analysis tool empowers you to respond appropriately and create meaningful connections with your customers.
By harnessing the power of AI to analyze customer sentiment, businesses can unlock a plethora of benefits. You can identify emerging trends, understand customer preferences, and uncover hidden opportunities for product innovation. This level of customer intelligence not only enhances the overall customer experience but also drives customer loyalty, advocacy, and ultimately, business growth.
In conclusion, customer sentiment analysis plays a vital role in understanding customer experiences, improving brand reputation, and anticipating market trends. By employing the right sentiment analysis techniques and leveraging tools like Rezo.ai, businesses can unlock valuable insights that drive customer satisfaction, loyalty, and overall success.
Frequently Asked Questions (FAQs)
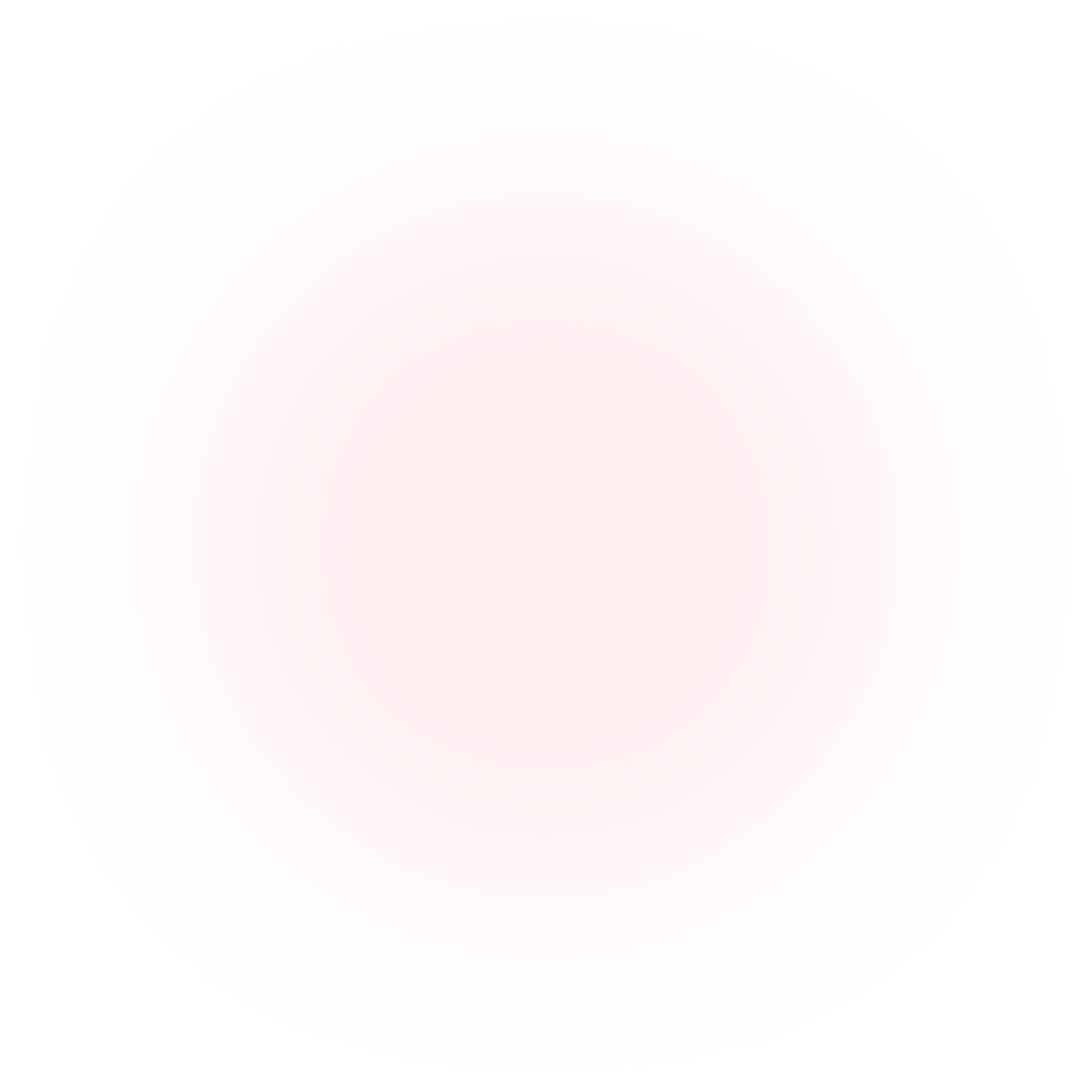