
6 Types of Agents in AI (Artificial Intelligence) for your Business

Artificial intelligence (AI) has grown fast over the years, giving rise to a diverse array of intelligent agents in AI that emulate human-like capabilities. Businesses are using these AI agents to automate procedures and minimize manual labor. These different types of agents in AI leverage cutting-edge technologies such as machine learning (ML) and natural language processing (NLP). AI agents are capable of automating and performing tasks ranging from simple appointment booking to complicated decision-making.
As per the Global State AI 2023 report, 87% of businesses believe that artificial intelligence (AI) and machine learning (ML) are important in achieving business goals. This can help in growing revenue, increasing operational efficiency, and boosting customer experience.
What are AI Agents?
AI agents, also known as artificial intelligence agents, are developed as autonomous programs or systems that simulate human-like intelligence and perform tasks or make decisions. The different types of agents in AI use Artificial intelligent algorithms and techniques to analyze data, learn from experience, and interact with their environment to achieve specific goals. The various types of intelligent agents in AI perceive their environment through sensors and act upon it through actuators.
Key characteristics of AI agents include
- Autonomy: They can operate independently, making decisions and taking action without constant human intervention.
- Adaptability: They can learn from data and adapt their behavior based on new information or changing circumstances.
- Interaction: They can interact with the environment, other agents, and users through various interfaces, such as text, voice, or visual inputs.
- Goal-Oriented: They are typically designed to achieve specific goals or objectives, whether it’s playing a game, providing customer support, or analyzing data.
Read more on Demystifying Myths about Virtual Agents
Types of Agents in AI for Your Business
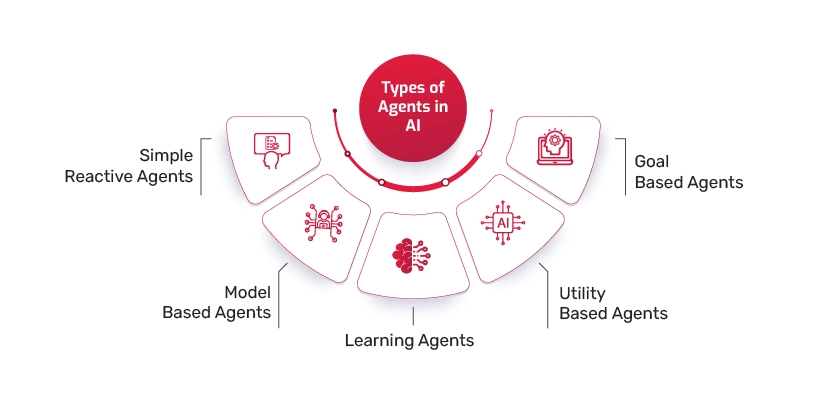
AI agents can vary in complexity and capabilities, ranging from simple rule-based systems to advanced machine-learning models. Let’s delve into various types of agents in AI, each tailored to specific functions and industries.
1. Simple Reactive Agents:
Simple reactive agents are one of the most basic types of agents in AI that come with the ability to provide basic automated assistance in business tasks. These agents make decisions based on predefined rules or conditions. They don’t have memory or the ability to learn from experience. Here are some use cases of simple reactive AI agents in business:
- Automated Customer Service Chatbots
- Appointment Scheduling
- Order Status and Tracking
- Basic Data Analysis and Reporting
- Virtual Shopping Assistants
- Language Translation and Localization
- Automated Surveys and Feedback Collection
- Basic Financial Transactions
- Inventory Management
- Lead Qualification
- Basic Data Entry and Record-Keeping
How do they work?
Simple reactive agents operate based on predefined rules and conditions. They analyze customer inputs, such as queries or requests, and provide immediate responses based on the available information. These agents excel at handling routine and straightforward tasks, ensuring swift and accurate customer support.
By leveraging machine learning and natural language processing, simple reactive agents can understand and interpret customer queries in real-time. This enables them to provide instant responses, reducing customer waiting times and increasing overall satisfaction.
Advantages of Simple Reactive Agents
- Real-time responsiveness: Simple reactive agents shine in delivering instant responses to customer queries. Customers no longer have to endure long wait times or navigate complicated automated menus. Quick and accurate replies create a seamless customer experience, enhancing satisfaction and fostering loyalty.
- Consistency and accuracy: These agents operate with predefined rules and conditions, ensuring consistent and standardized responses. This minimizes the risk of human errors or inconsistencies that can impact the customer experience. Customers can rely on the accuracy and reliability of simple reactive agents for reliable service.
- Cost and resource efficiency: Simple reactive agents streamline contact center operations by handling routine inquiries autonomously. This reduces the workload on human agents, allowing them to focus on more complex and specialized tasks. The result? Improved resource allocation, reduced operational costs, and optimized productivity.
- Scalability and 24/7 availability: As businesses grow and expand, so does the demand for customer support. Simple reactive agents can effortlessly scale to handle increased customer volumes without compromising responsiveness. Their virtual nature enables them to provide round-the-clock support, ensuring customers are assisted whenever they need it.
Disadvantages of Simple Reactive Agents
- Limited problem-solving capabilities: While simple reactive agents excel in handling routine queries, their scope is often limited to predefined rules. They may struggle with complex or unique customer scenarios that require creative problem-solving or in-depth analysis. Human agents may still be necessary for handling such situations.
- Dependency on accurate data and knowledge base: Simple reactive agents rely heavily on accurate data and a robust knowledge base. Ensuring the agents have access to up-to-date and relevant information is crucial for delivering accurate responses. Maintaining and updating the knowledge base can be challenging and time-consuming.
- Lack of personalization: Simple reactive agents prioritize efficiency and speed, which may come at the cost of personalization. These agents may not possess the ability to establish emotional connections or understand nuanced customer preferences. Augmenting them with contextual information or transitioning to more advanced AI models may be necessary for achieving a higher level of personalization.
Also Read: How AI Voice Bots are Helping Telecommunications Industry in Dormant Reactivation
2. Model-Based Agents
Model-based AI agents are more competent. These agents maintain an internal representation of the world and use it to optimize processes, strategies, and decisions by simulating different scenarios before taking action. These agents come with the ability to consider multiple actions and predict the outcomes of those actions. Here are some use cases of model-based agents in business:
- Demand Forecasting
- Resource Allocation
- Pricing Strategy Optimization
- Portfolio Management
- Marketing Campaign Planning
- Risk Assessment and Management
- Healthcare Treatment Planning
- Loan Approval and Credit Scoring
- Employee Performance Prediction
How do they work?
Model-based agents in AI rely on explicitly built models of the environment to make decisions and take actions. These agents create an internal representation or simulation of the environment, which they use to predict the outcomes of different actions.
They use this model to simulate various scenarios and evaluate the potential consequences of their actions. By analyzing the predicted outcomes, model-based agents can make informed decisions based on the desired goals or objectives.
Advantages of Model Based Agents
- Improved decision-making: Model-based agents excel in making well-informed decisions as they have a detailed understanding of the environment through their built models. This allows them to anticipate and account for potential outcomes and make better choices.
- Simulation and planning capabilities: By simulating different scenarios, model-based agents can plan ahead and assess the consequences of potential actions. This enables them to select the most optimal course of action and avoid undesirable outcomes.
- Flexibility and adaptability: Model-based agents can quickly adapt to new environments or changes in existing environments by updating their internal models. This flexibility allows them to respond effectively to dynamic situations and adjust their strategies as needed.
- Reduced reliance on large datasets: Unlike some other AI approaches, model-based agents require less reliance on a massive amount of training data. The focus is on building an accurate model rather than solely relying on a vast dataset for training.
Disadvantages of Model Based Agents
- Challenges in accurate model building: Constructing an accurate model of the environment can be complex and time-consuming. It requires a deep understanding of the domain and may involve handling uncertain or incomplete information.
- Limited generalization beyond the model: Model-based agents heavily rely on the accuracy and completeness of the built models. If the models do not accurately capture the complexity of the environment, the agent's decisions may not generalize well beyond the model.
- Computational complexity and resource requirements: Model-based agents can be computationally intensive, particularly when simulating complex environments or conducting extensive planning. This may require significant computational resources and processing power.
- Inability to handle unknown or unpredictable environments: Model-based agents may struggle to perform optimally in environments that are highly unpredictable or involve unknown factors. Their reliance on accurate models makes it challenging to operate in such uncharted territories.
Also Read: How Conversational Analytics Can Help Banks Better Understand Their Customers
3. Learning Agents
Learning agents are the types of AI agents that possess the ability to learn from data and experiences to improve their performance over time. These agents use machine learning techniques to adapt and make better decisions as they gather more information. Here are use cases of learning agents in business:
- Customer Segmentation
- Personalized Recommendations
- Fraud Detection
- Churn Prediction
- Sentiment Analysis
- Dynamic Pricing
- Inventory Management Optimization
- Language Translation Improvement
- Quality Control
- Employee Training and Skill Enhancement
- Predictive Maintenance
How does it work?
Learning agents in AI are designed to acquire knowledge and improve their performance based on experience. These agents employ various learning techniques such as supervised learning, unsupervised learning, and reinforcement learning to adapt and optimize their behavior.
Through supervised learning, learning agents are trained using labeled examples to recognize patterns and make predictions. Unsupervised learning enables agents to explore data and identify hidden patterns and structures without prior knowledge. Reinforcement learning involves agents interacting with an environment, receiving rewards or penalties based on their actions, and learning optimal strategies through trial and error.
Advantages of Learning Agents
- Adaptability and flexibility: Learning agents have the ability to adapt to new situations and adjust their behavior based on changing environments. They can continuously learn from new data and improve their performance over time.
- Automation and efficiency: These agents can automate tasks by learning from large datasets, thereby reducing the need for manual intervention. They can process and analyze data at a faster rate than human counterparts, leading to improved efficiency.
- Decision-making based on data: Learning agents make decisions based on data-driven insights, reducing bias and human errors. They can process and analyze vast amounts of data to uncover patterns and make informed decisions.
- Continuous improvement: Learning agents have the capability to continuously improve their performance through iterative learning cycles. They can learn from mistakes and adjust their strategies to achieve better outcomes.
Disadvantages of Learning Agents
- Data dependency and quality: Learning agents heavily rely on the quality and quantity of data they receive for training. Insufficient or biased data can lead to inaccurate models and flawed decision-making.
- Computational complexity and resource requirements: Training learning agents can be computationally intensive and require significant computational resources. Complex models or large datasets may necessitate high-end hardware or cloud-based computing resources.
- Ethical considerations and bias: Learning agents can inherit biases from the data they are trained on, potentially leading to biased decision-making. Care must be taken to ensure fairness and avoid discriminatory outcomes.
- Interpretability and transparency: Some learning algorithms, such as deep neural networks, can be difficult to interpret, making it challenging to understand the reasoning behind their decisions. This lack of transparency can limit trust and acceptance in certain domains.
4. Utility-Based Agents
Utility-based AI agents make intelligent decisions by focusing on maximizing the measure of utility to maximize a specific goal. These agents consider not just the likelihood of outcomes but also the utility or value associated with those outcomes. Here are some use cases of utility-based agents for business:
- Investment Portfolio Management
- Product Feature Selection
- Supply Chain Optimization
- Marketing Budget Allocation
- Staff Scheduling
- Energy Consumption Management
- Customer Segmentation for Promotions
- Project Prioritization
- Vendor Selection
- Inventory Replenishment
How does it work?
Utility-based agents in AI make intelligent decisions by focusing on maximizing the measure of utility to achieve a specific goal. These agents consider not just the likelihood of outcomes but also the utility or value associated with those outcomes.
They employ advanced algorithms and techniques to analyze data, evaluate potential outcomes, and calculate the utility of each decision. By comparing the expected utilities of different actions, utility-based agents can determine the most optimal course of action to take in a given situation.
Advantages of Utility Based Agents
- Optimal decision-making: Utility-based agents excel in making optimal decisions by considering the utility of different outcomes. This ensures that actions are chosen based on their potential to maximize desired goals.
- Flexibility and adaptability: These agents are well-suited for dynamic environments as they can adapt and adjust their decisions based on changing circumstances or new information. This enables them to respond effectively to evolving situations.
- Customization and personalization: Utility-based agents can be customized to reflect specific preferences, priorities, and constraints. This allows businesses to tailor their decision-making processes and strategies to align with their unique requirements.
- Efficiency and resource optimization: By focusing on maximizing utility, these agents can efficiently allocate resources, allocate budgets, and prioritize tasks. This leads to improved resource utilization and cost efficiency.
Disadvantages of Utility Based Agents
- Complexity and implementation challenges: Implementing utility-based agents requires significant computational power, data analysis capabilities, and expertise. Developing and fine-tuning the utility functions and decision-making models can be complex and time-consuming.
- Dependency on accurate utility functions: The effectiveness of utility-based agents heavily relies on the accuracy and reliability of utility functions. If the utility functions are not well-defined or do not accurately capture the desired goals, the decision-making process may lead to suboptimal outcomes.
- Limited applicability: Utility-based agents are best suited for scenarios where the goals can be quantified and translated into utility measures. In situations where goals are subjective or difficult to quantify, utility-based approaches may not be suitable.
- Incomplete information and uncertainty: Utility-based agents rely on available information to calculate utilities and make decisions. In cases where information is incomplete or uncertain, the accuracy of utility calculations and the subsequent decision-making process may be affected.
Read More: Generative AI Vs Predictive AI: Unveiling the Differences
5. Goal-Based Agent
Goal-based AI agents plan and execute actions to achieve specific & desired goals and objectives. This intelligent agent in AI possesses the ability to use their knowledge and reasoning abilities to determine the best course of action that will bring them closer to the desired outcomes. Here are some use cases of goal-based agents for business:
- Sales and Marketing Optimization
- Customer Service Enhancement
- Supply Chain Management
- Financial Decision-Making
- Human Resources and Talent Management
- Data Analytics and Insights
- Process Automation
- Risk Management
- Product and Service Innovation
How Does it Work?
Goal-based agents use several techniques such as state-space search, rule-based reasoning, utility-based evaluation, and optimization algorithms to make decisions that align with preset objectives. These agents first establish the initial state of the environment, determine the goal state, and then generate a sequence of actions that will take them from the initial to the goal state.
The agent continuously evaluates the potential outcomes of its actions, analyzing how close each action takes it to its target and then selects a series of actions that provide the best chances of achieving the desired goal.
Advantages of Goal Based Agents
- Accurate and Efficient Decision-Making Process: Goal-based agents are designed to achieve specific purposes. Their use of AI-powered technologies ensures that their decisions are made available with high accuracy while remaining highly efficient.
- Flexibility and Adaptability: Goal-based agents can adapt to varying environments and changes in the environment parameters. Due to their versatile nature, goal-based agents can learn and modify its behavior according to the user's needs.
- Real-time Evaluation of Outcomes: With this AI-powered technology, goal-based agents can assess every potential outcome of an action and make decisions in real-time. This feature ensures that every possible scenario is considered before an action is taken, which helps ensure optimum outcomes.
- Improved Resource Management and Cost Efficiency: Goal-based agents can optimize the computational resources used, which ensures cost-efficient operation in any given environment, leading to better resource management for the user.
Disadvantages of Goal Based Agents
- Requires a detailed understanding of the environment: For a goal-based agent to make real-time strategic decisions, developers need to have an in-depth knowledge of the environment in which the agent is operating.
- Limited Focus: Goal-based agents focus explicitly on the objective defined in the environment. Therefore, if other factors come into play that are not related to the defined objective, the agent may not be able to recognize them.
- Can be time-consuming: Developing, training, and fine-tuning goal-based agents takes a lot of resources and time before establishing meaningful results.
- Lack of Creativity: Goal-based agents are designed to seek optimum outcomes for objectives they are hardcoded to achieve, which lack creativity compared to human thought processes. Therefore, they may have challenges solving unique and unfamiliar problems since they need to follow preprogrammed rules and conditions.
Read More: Top Conversational AI Trends in 2023
6. Hierarchical Agent
A Hierarchical Agent is an advanced AI solution that helps businesses manage and optimize complex operations across various levels. It enables efficient allocation of tasks and responsibilities based on skill levels and proficiency. With Hierarchical Agents, businesses can monitor team performance, streamline communication, and boost productivity. Here are some use cases of hierarchical agents for business:
- Sales and Marketing Optimization
- Customer Service Enhancement
- Supply Chain Management
- Financial Decision-Making
- Human Resources and Talent Management
- Data Analytics and Insights
- Process Automation
- Risk Management
- Product and Service Innovation
How Does it Work?
Hierarchical agents are structured using a hierarchical model that consists of various levels or modules. Each level is responsible for a specific subtask and communicates with higher and lower levels to exchange information and achieve the overall goal. The lower-level agents handle detailed and specific tasks, while higher-level agents coordinate and control the lower-level agents.
This hierarchical structure allows agents to distribute workload, increase efficiency, and handle complex problems by breaking them down into simpler components. Communication between levels facilitates information sharing, feedback, and decision-making, enhancing overall performance.
Advantages of Hierarchical Agents
- Improved Problem-Solving: Hierarchical agents excel at managing complex tasks by breaking them down into smaller, more manageable subtasks. This approach simplifies problem-solving, increases efficiency, and allows for quicker resolution of complex issues.
- Flexible and Scalable: The modular nature of hierarchical agents makes them highly adaptable and easily scalable. New modules can be added or modified to accommodate changing requirements, enabling the system to evolve and handle a wide range of tasks without significant restructuring.
- Efficient Resource Allocation: By distributing tasks among different levels, hierarchical agents optimize resource allocation. Each level can focus on its specific task, utilizing resources effectively and avoiding redundant efforts. This leads to improved performance and resource utilization.
- Better Decision-Making: With hierarchical agents, decision-making becomes a collective effort. Higher-level agents can receive input and feedback from lower-level agents, leading to more informed and comprehensive decisions. This collaborative approach enhances the quality and accuracy of decision-making processes.
Disadvantages of Hierarchical Agents
- Increased Complexity: The hierarchical structure of these agents adds complexity to the system design and implementation. Managing the interactions between multiple levels and ensuring effective communication can be challenging, requiring careful planning and design.
- Dependency on Information Flow: Hierarchical agents heavily rely on the flow of information between different levels. If there are gaps or delays in information exchange, it can impact the overall performance and decision-making capabilities of the system.
- Limited Flexibility in Dynamic Environments: Hierarchical agents may face difficulties in adapting to rapidly changing or unpredictable environments. The predefined structure and modules may not be able to handle unforeseen situations, requiring system modifications or alternative approaches.
- Initial Development and Integration Efforts: Designing and implementing a hierarchical agent system requires significant upfront efforts in terms of development and integration. The process involves defining the hierarchy, creating modules, and ensuring seamless communication between levels, which can be time-consuming and resource-intensive.

AI agents Vs. Human Agents
AI agents can increase work efficiency and precision in decision-making by five folds. Various types of agents in AI are now growing their capabilities and helping human agents. They can now aid in a diverse range of business tasks, from simple customer query resolution to complex decision-making and problem-solving in businesses. AI’s computational prowess is leading to a paradigm shift that has far-reaching implications across various domains and industries.
The development and adoption of AI agents has introduced significant shifts in several aspects of the workforce. While machines cannot yet fully replace humans, AI agents can perform certain tasks and activities more efficiently, accurately, and consistently than their human counterparts. Here are some of the key differences between AI agents and human agents:
Advantages of AI Agents:
- Efficiency: AI agents can handle repetitive workloads and can provide 24/7 service without breaks. This feature promotes consistency and minimizes the potential for errors, increasing productivity.
- Accuracy: AI agents analyze data and execute commands with high accuracy, as they are bound to specific rules programmed into them.
- Faster Decisions: AI agents can analyze data and make decisions in real-time or within seconds, eliminating the need for humans to process such a high volume data.
- Cost-Saving: Since AI agents can work endlessly, they can save costs over time in operations that require long hours. This feature makes them an attractive option for businesses with high operational costs.
Advantages of Human Agents:
- Empathy: Human agents can readily discern human emotions and provide appropriate responses and assistance that to an extent, cannot be replicated by machines.
- Critical Thinking: Humans have the ability to think creatively and critically, which allows them to solve complex problems efficiently and using varied approaches.
- Flexibility: Humans have the ability to adapt to unforeseen circumstances, which makes them a better option for multiple tasks within a context.
- Contextual Understanding: Humans have a better experience in understanding nuances of human society context and handling delicate situations that require in-depth reasoning skills.
Where is the balance?
While AI agents have the potential to outperform humans in specific tasks, there are shared capabilities and limitations. While humans will likely continue playing a vital role in the workforce, AI agents are expected to offer improved support services to humans, enhancing the efficiency and effectiveness of human work.
To get the full benefits out of AI agents and human agents, we need to understand where each is best used. AI agents are better suited for routine, high-volume tasks with limited cognitive requirements, while humans excel at tasks that need contextual understanding, empathy, and critical thinking. Combining the areas in which human and AI agents excel may allow businesses to deliver fast and optimized solutions, while allowing both AI agents and human agents to provide the utmost quality service to customers and companies alike.
Transform your Business with AI Agents of Rezo.ai
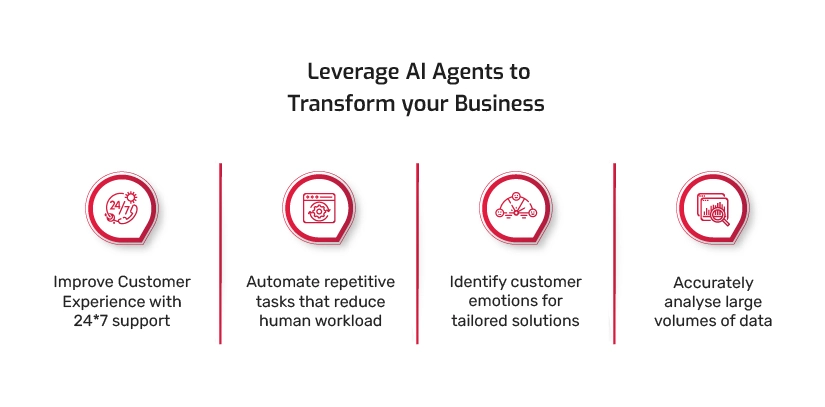
The AI agents of Rezo.ai can transform your business in various aspects. Rezo.ai is an AI-powered CX cloud for enterprises equipped with state-of-the-art features of Machine learning and natural language processing. The different types of agents in AI of Rezo.ai can be leveraged for the following:
- Better CX: Provide instant and personalised customer support 24/7. Answer common queries, guide customers through processes, and offer product recommendations, enhancing overall customer satisfaction and engagement.
- Automate mundane tasks: Rezo’s Engage AI takes over repetitive tasks such as data entry & appointment scheduling. This frees up human resources to focus on more strategic and creative tasks.
- Better Tone Analysis: Analyse text and voice interactions to identify customer emotions and sentiments to tailor their responses, leading to more empathetic and effective communication.
- Cost-effective: By Automating tasks and processes, Rezo’s AI agents help reduce the need for manual labour, leading to cost savings in terms of human resource allocation and time efficiency.
- Analyze Data: Rezo’s Analyse AI processes analyses large volumes of data quickly and accurately, extracting valuable insights, trends, and patterns to make data-driven decisions.
Witness the future of business today – Request a Demo now!
FAQs on Types of Agents in AI
Frequently Asked Questions (FAQs)
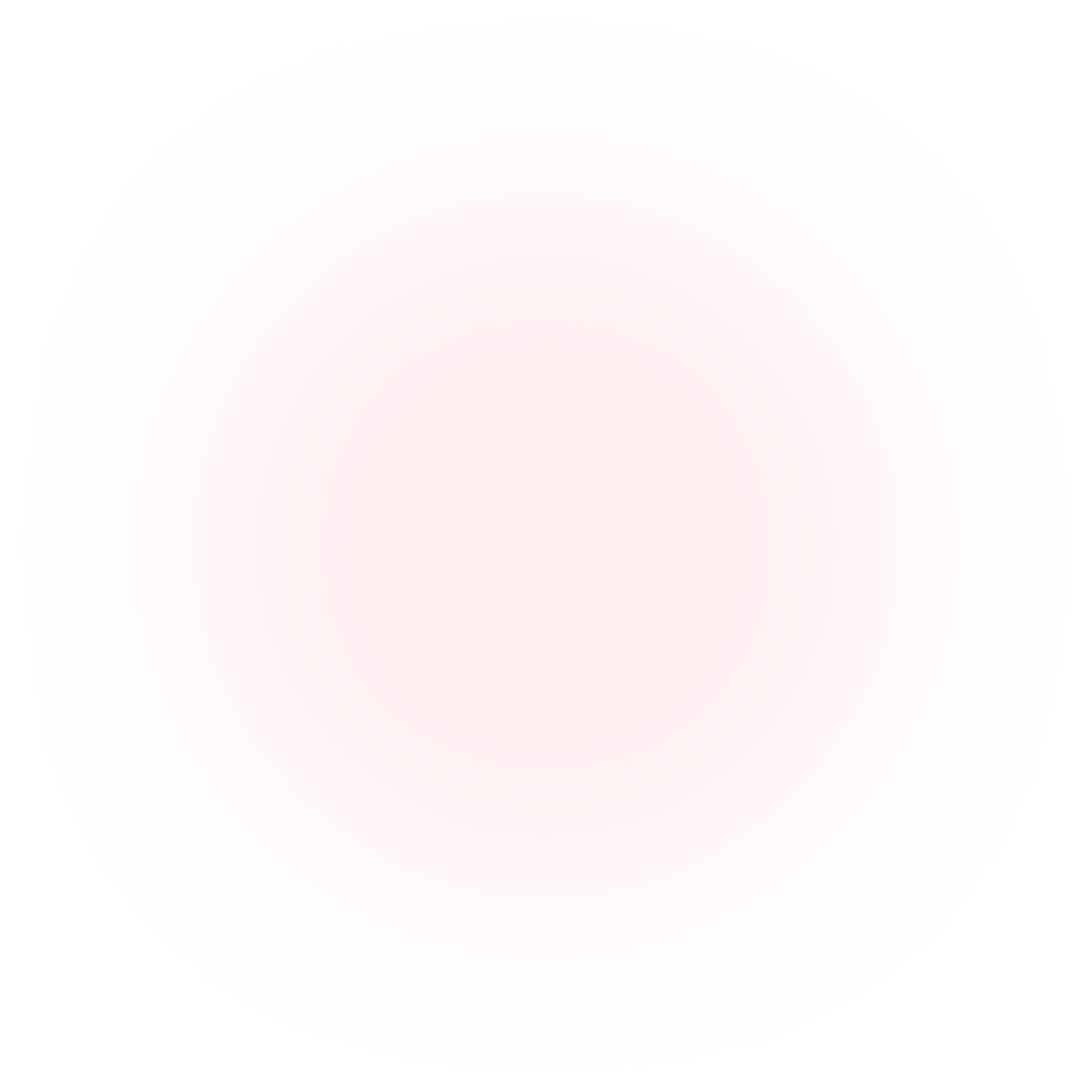